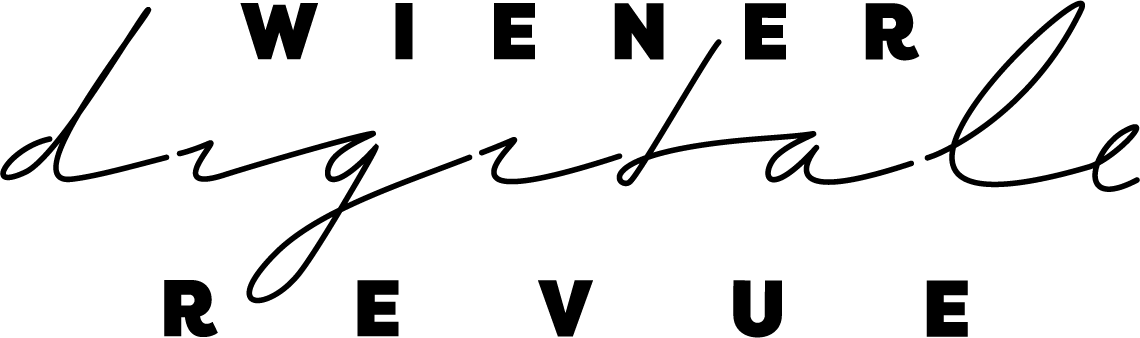
Zeitschrift für Germanistik und Gegenwart
Karl Wolfgang Flender
From Crime Scene Investigation to Pattern Recognition
Lizenz:
For this
publication, a Creative Commons Attribution 4.0 International license
has been granted by the author(s), who retain full
copyright.
Link
Wiener Digitale Revue 5 (2024)
www.univie.ac.at/wdrAbstract
Top of pageFull text
Top of page
The classic detective figure has become obsolete in the age
of Big Data and Pre-Policing. Crime literature is facing a turning
point.1
When torture was finally abolished in the 18th century, ending practices like quartering, breaking on the wheel and the use of thumbscrews, a new method was needed to convict suspected criminals. Instead of a coerced confession, courts suddenly demanded evidence for the crime. The figure of the modern investigator stepped onto the scene – and with it emerged the crime narrative as we know it today: Case histories with the perpetrator at the center evolved into tales focusing on the work of the investigator. Fast forward to the beginning of the 21st century: Today we are experiencing a revolution in criminalistics not seen since the late Enlightenment. The digital age is coming for the detectives. But crime literature seems to be sleeping through this upheaval.
Whether Miss Marple traces a murderer’s footprints in a rose garden between crochet sessions and her five o’clock tea, whether Sherlock Holmes solves puzzles while playing the violin, or whether Philip Marlowe stumbles through rainy L.A. with a cigarette in the corner of his mouth – the logic is always the same: The investigator, as the reader’s proxy, collects clues, interprets them, and assembles the traces into ever-new hypotheses. Carlo Ginzburg calls this the “evidential paradigm”, which is not only the principle underlying most crime literature, but also the diagnostics of modern medicine or Freud’s psychoanalysis, and serves as a model for all sciences focused on individual cases (see Ginzburg 1989: 96). Detection, as an interpretative act, uncovers a complex reality in seemingly insignificant empirical data: It is the minor and involuntary observations that betray the perpetrators, tiny details that only catch the eye of a detective.
Ginzburg names “instinct, insight, intuition” as the intangible elements of this type of knowledge production (ibid.: 125), the rules of which cannot be pinned down, which is why crime literature was populated by idiosyncratic geniuses right from the start. As stated in the first sentence of Edgar Allan Poe’s The Murders in the Rue Morgue, which established the detective genre in 1841: “The mental features discoursed of as the analytical, are, in themselves, but little susceptible of analysis” (Poe 2006: 129). Poe’s genius private investigator Dupin, for example, practices “ratiocination” – a deductive “method” based on keen observation, understanding of human nature, and openness to the absolutely improbable. The Murders in Rue Morgue is a case in point: In the end, the murderer turns out to be an ape.
Since the Enlightenment, the crime novel in its various forms has thus been underpinned by an epistemological model, a particular approach to perceiving the world, that concentrates on details and their interpretation. This approach manifests in a dual narrative structure: A detective’s story of investigation that reconstructs the story of a crime through the interpretation of clues.
Until today. In the digital era, it’s not just the perpetrator’s traces that are collected at the crime scene after the fact, but rather the traces of innocent people, always and everywhere. Amazon, Facebook, and Google treat us all like criminals when they apply standard profiling techniques out of the modern criminalistics playbook: tracking movement data, analyzing behavioral patterns, and identifying relationship networks to catalog their users’ desires and fears, offer them products, or influence their behavior in elections. Whereas surveillance and punishment used to be the realm of the state, nowadays IT companies have assumed control over the subjects. Alternatively, individuals discipline themselves in a form of proactive compliance, following the motto: ‘I’d better not google that.’
Not only do the classic detectives have competence issues when their field is suddenly absorbed by Californian start-ups, but the ubiquitous datafication also threatens the very foundation of their profession: the secret at the heart of every criminal case. After all, what becomes of the secret when everything and everyone is digitally grid-searched, scrutinized, and analyzed? When all motives, desires, and drives are denounced by data, when every step is predictable, how can gangsters still plot conspiracies or carry out bank robberies? Can’t we just mothball the detectives then? Why do we still need lone wolves when everyone voluntarily hands their fingerprint to their smartphone? Why still carry out secret surveillance operations at night when every face on the street is recognized by an algorithm? If we're already permanently monitored by our Amazon Echo, why still smuggle bugs into hotel rooms?
The simplest solution for fiction writers is to update the detective a bit. In the brave new world, other virtues are in demand: The investigator becomes a hacker or data analyst, as in the American TV series numb3rs, where algorithms developed by a genius mathematician lead to the capture of serial offenders. But this conventional update ignores the much more fundamental problem of the detective in the digital present and draws hardly any narrative conclusions from it: Computer-aided analytics differs starkly from clue-driven investigation. Knowledge today is no longer created by interpreting a limited number of clues but by pattern recognition within vast databases. While clue reading is a qualitative method interested in the individuality of cases, situations, and people, the new methodology is quantitative. Everything that cannot be statistically evaluated is disregarded: flair, judgement, instinct. Before: human interpretation. Today: machine correlation. Big data instead of the devil in the details. The causal linking of traces to a chain of evidence from which a story emerges turns into the statistical, synchronous correlation of data points – and thus the evidential paradigm and with it the dual nature of crime fiction narratives is replaced. There is no longer a narrative, only calculation.
Big data analytics is also incompatible with the reconstructive approach of the investigator for another reason: Instead of drawing conclusions for the present from past data, it increasingly focuses on predicting the future under the label of “predictive policing”. Philip K. Dick already presented the clairvoyant vision of such a “pre-crime” system in 1956 with The Minority Report, in which murders could be foreseen and stopped with the help of mutants, not only inspiring the imagination of his readers but also that of criminologists – who have implemented pre-crime in reality in a far more dystopical sense than in fiction: Suspects are no longer convicted because their characteristics match police databases (as with fingerprints, saliva samples, mugshots), or does the police evaluate data sets of already convicted offenders to calculate preventive recidivism risk. Rather, in big data policing, people are classified as suspicious through speculative statistics because individual data points of their profile correlate with environmental factors, behavioral patterns, or data points of criminals (comparable to Amazon’s credo: “Customers who bought a knife set and a wire noose also...”).
This is called “guilt by association” (Cramer 2018: 31): Just because you live in this neighborhood, share GPS coordinates with those people, or have similar routines, you become suspicious. Not because you have committed a crime, but because the algorithm indicates that you could commit one in the future. Studies in the U.S., where such programs are already in use, show not only that they (so far) have a lousy success rate but also that people with black skin are twice as likely to be wrongly classified as future violent offenders than people with white skin (ibid.). This does not deter police forces and software manufacturers from marketing such algorithms as more objective than human investigation while the programmers’ prejudices are reflected in the programming. The reactionary positivism of start-up culture is thus very likely to get along rather well with today’s fascists. By the way, the codes that govern public affairs are not for the public: they are kept under lock and key as a trade secret.
Big data thus not only does away with the evidential paradigm, but with predictive policing, criminalistics also switches from a past to a future orientation. Thus, the fundamental structure of crime literature – the dual structure of investigation and crime story –, as it has existed more or less since the late Enlightenment with its shift from the forced confession model to the investigation model, is bid farewell. With data correlation, the detective novel itself becomes questionable.
Contemporary crime literature that does not reflect this state of affairs is naive. Or unrealistic. Not to mention: negligent. Because it deals with a world of yesterday. It’s cozy and familiar, no doubt, and it’s an escape from the challenges of the present world. In this regard, the standard crime novel is no different from the Sunday crime show: the same faces, the same plot patterns, and occasionally, we take a quick restroom break. It's a form of historical storytelling. No longer contemporary.
Therefore, crime literature in the digital age will have to reinvent itself. The current transformation of criminalistics affects its epistemological and narrative substance. If the previous model of crime literature was the chain of evidence, the new model is the database, in which data points are synchronously connected to patterns by algorithmic correlation – and thus is not easily transferable to the classic, diachronic-linear mode of presentation of literature. This demands a new, experimental storytelling that goes far beyond what, for example, the (moderately clever) show numb3rs has experimented with in terms of data analysis, mathematics, statistics presentation, or non-linearity. And it requires a stance: Does the new crime novel reproduce the totalitarian rhetoric of start-ups, politics, and the police apparatus – or does literature try to challenge the big data aficionados? Because, as Poe already knew: “[T]o calculate is not in itself to analyze.” (Poe 2006 [1841]: 130)
Otherwise, the detective novel will become obsolete like the old heroes of the night. The detectives with their fedoras are currently fighting their last battle. To put it in the language of the genre: It is a matter of life and death.
Bibliography
Top of page- Cramer, Florian (2018): Crapularity Hermeneutics: Interpretation as the Blind Spot of Analytics, Artificial Intelligence, and Other Algorithmic Producers of the Postapocalyptic Present, in: Clemens Apprich/Wendy Hui Kyong Chun/Florian Cramer/Hito Steyerl (ed.): Pattern Discrimination. Minneapolis: meson press, pp. 23–58.
- Poe, Edgar Allen (2006 [1841]): The Murders in the Rue Morgue, in: The Collected Works of Poe, Volume I, San Diego: Icon, pp. 129–164.
- Ginzburg, Carlo (1989 [1986]): Clues. Roots of an Evidential Paradigm, in: Clues, Myths and the Historical Method, translated by John and Anne C. Tedeschi. Baltimore: The Johns Hopkins University Press, pp. 96–125.